Situational Awareness for an autonomous inland vessel
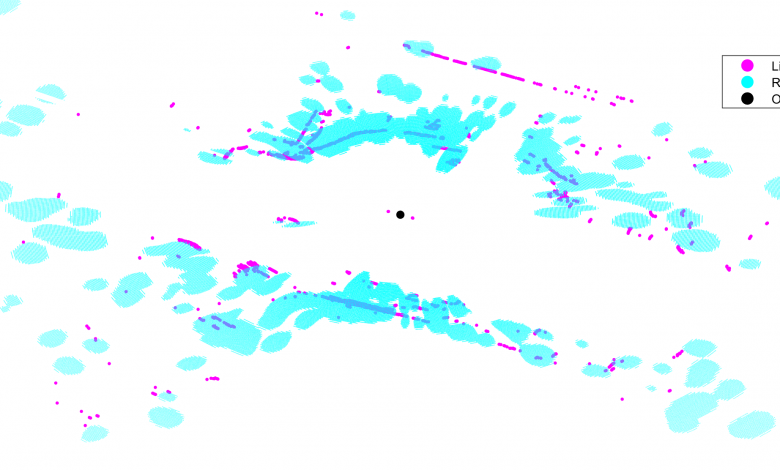
A key task for any captain or crew of a vessel is maintaining a correct understanding of the current and future situation of the vessel, i.e., having good situational awareness. Therefore, a key capability of an autonomous vessel is to maintain a similar or better degree of situational awareness compared to a manned vessel. For this, sensors are required. Since sensors have different strengths and weaknesses, it is desirable to combine data from several sensors to build an overall system that is better than a single sensor. This process is known as ‘sensor fusion’.
Image: A Radar and LiDAR scan of an urban waterway. Taken from milliAmpere2 operating in the Trondheim canal [1].
An example of the benefits of sensor fusion is LiDAR and Radar fusion. The image shows a scan of a LiDAR and a Radar on top of one another, and this demonstrates the benefits and drawbacks of both sensors. We can see that the Radar has a longer range of detection but is less accurate in its measurements as compared to a LiDAR, where we can discern greater details of the shoreline and moored vessels along it. To utilize the strengths of both sensors, Radar can be used for initially detecting targets further away and a LiDAR can be used to give an exact position of objects that are closer.
Other important sensors are cameras, which can be used to detect and recognize specific objects. In addition, we also need to know the position and speed of our own ship, which can be provided by a GPS system combined with an IMU (Inertial Measurement Unit). In the maritime sector we also have access to AIS (Automatic Identification System), with which other larger ships can broadcast their position and speed [2]. A data source of particular importance for inland waterways is IENC (Inland Electronic Navigational Charts) which contains information about the location of the shoreline and static obstacles in the waterway. In addition, we also need to know the position and speed of our own ship, which can be provided by a GPS system combined with an IMU.
These sensors provide the needed data, but we also need to understand what the data gathered means. For this, sensor fusion algorithms are required that combine data from these different sensors to extract relevant information. These algorithms could be very complex, but broadly speaking they need to have the ability to determine where the measurements are coming from. For instance, in the picture above, which measurements are coming from moving vessels, and which are from land or static objects? If there are multiple moving vessels it also needs to establish which vessel the measurement is coming from. The aim is to estimate the position, speed, heading and size of other vessels, the position of static obstacles and land as well as the position of our own vessel in the waterway so that vessel can be navigated in a safe manner.
While there are already algorithms that address the tracking of other vessels, as well as localization of a vessel, for navigation in an inland waterway these algorithms need to be combined. This is what I hope to achieve with my research.
An article by Martin Baerveldt.
References:
[1] E. F. Brekke et al., “milliAmpere: An Autonomous Ferry Prototype,” J. Phys.: Conf. Ser., vol. 2311, no. 1, p. 012029, Jul. 2022, doi: 10.1088/1742-6596/2311/1/012029.
[2] S. Thombre et al., “Sensors and AI Techniques for Situational Awareness in Autonomous Ships: A Review,” IEEE Transactions on Intelligent Transportation Systems, vol. 23, no. 1, pp. 64–83, Jan. 2022, doi: 10.1109/TITS.2020.3023957.