Applying Vision-Language Models in Autonomous Driving: Can the Insights Be Transferred to Autonomous Vessels in Inland Waterways?
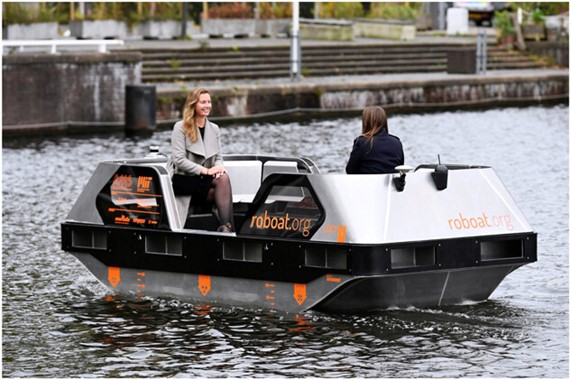
Vision-Language Models (VLMs), due to their exceptional capabilities in environment comprehension and multimodal information processing, have garnered significant attention in the field of Autonomous Driving (AD). They offer innovative solutions to complex scene interpretation and interactive navigation challenges in AD [1].
Figure 1. Roboat, a self-driving boat, was being tested at the AMS Institute. [2]
Meanwhile, research on autonomous vessels has increased dramatically during the last decade. Sensors and artificial intelligence (AI) technologies are increasingly used by vessels to navigate, maneuver, and avoid collisions [3]. Notable progress is evident, as exemplified by the Roboat III prototype, which is currently being tested for an autonomous water taxi task at the AMS Institute (see Fig 1). However, compared to autonomous driving systems, the development of autonomous vessels is still in its nascent stages. To the best of my knowledge, there is no existing research that applies Large Language Models (LLMs) and Vision-Language Models (VLMs) specifically to autonomous vessels in inland waterways [2].
This blog will briefly discuss some popular large models, such as ChatGPT-4V and Contrastive Language-Image Pre-training (CLIP) in the context of VLMs, focusing on their downstream applications in AD. Additionally, it will explore the potential of applying these models to autonomous vessels.
Figure 2. Results on the ability to understand the traffic light. [4]
Figure 3. Results that identify the weather in which the vehicle is driven. [4]
GPT-4V: Recognizing traffic signals and weather.
Recent studies have extensively evaluated GPT-4V’s performance across a range of autonomous driving scenarios [4]. As shown in Fig 2, GPT-4V demonstrates excellent traffic signal recognition ability, clearly recognizing red and yellow traffic lights, even in nighttime situations. In addition, the weather has a profound impact on driving behavior, and accurate weather recognition can help improve driving safety to a certain extent. Figure 3 illustrates GPT-4V’s capability to comprehend various weather conditions, demonstrating its potential to enhance driving safety. Of course, the study also found some limitations of GPT-4V, which can exhibit confusion in some complex situations.
Figure 4. Qualitative results of annotation-free semantic segmentation on nuScenes dataset. [5]
CLIP:For 3D scene understanding.
Another milestone VLM model, CLIP, achieves zero-shot transfer capability by capturing language-related image feature representations and training with many images and texts through contrastive learning. CLIP2Scene, a study on Vision Language Models in Autonomous Driving and Intelligent Transportation Systems, makes it possible to apply VLM to the autonomous driving 3D scenes. This framework is built on CLIP. It uses semantic and spatiotemporal consistency regularization to pre-train 3D networks [5]. Tests conducted on multiple datasets have shown significant improvements in 3D semantic segmentation.
Discussion:Can the Insights Be Transferred to Autonomous Vessels in Inland Waterways?
The VLMs in Object Detection of Autonomous Vessels on Inland Waterways offer a promising avenue, especially when considering the unique challenges and limited data availability in this domain [6]. This approach could enable the recognition of crucial elements in the maritime environment, such as weather conditions, navigational aids, and traffic signals unique to inland waterways.
However, the distinctive nature of inland waterway environments, markedly different from standard road scenarios, raises concerns about the limitations of zero-shot methods. These environments are characterized by their dynamic nature, including varying water currents, floating objects, and dynamic traffic patterns, which present challenges that may not be fully captured in the existing zero-shot methods. This gap necessitates further investigation into the adaptability and robustness of zero-shot learning in such specific contexts.
Moreover, the ‘black-box’ nature of zero-shot models introduces inherent uncertainties regarding their predictability and reliability. Safety and reliability are paramount in autonomous navigation, and any uncertainty can pose significant risks. This concern underscores the need for ongoing research to better understand, evaluate, and possibly mitigate the risks associated with the deployment of these models in safety-critical maritime applications.
An article by Zhongbi Luo.
References
[1] Zhou X, Liu M, Zagar B L, et al. Vision language models in autonomous driving and intelligent transportation systems[J]. arXiv preprint arXiv:2310.14414, 2023.
[2] Wang W, Fernández‐Gutiérrez D, Doornbusch R, et al. Roboat III: An autonomous surface vessel for urban transportation[J]. Journal of Field Robotics, 2023, 40(8): 1996-2009.
[3] Negenborn R R, Goerlandt F, Johansen T A, et al. Autonomous ships are on the horizon: here’s what we need to know[J]. Nature, 2023, 615(7950): 30-33.
[4] Wen L, Yang X, Fu D, et al. On the road with GPT-4V (ision): Early explorations of visual-language model on autonomous driving[J]. arXiv preprint arXiv:2311.05332, 2023.
[5] Chen R, Liu Y, Kong L, et al. CLIP2Scene: Towards Label-efficient 3D Scene Understanding by CLIP[C]//Proceedings of the IEEE/CVF Conference on Computer Vision and Pattern Recognition. 2023: 7020-7030.[6] Billast M, Janssens R, Vanneste A, et al. Object Detection To Enable Autonomous Vessels On European Inland Waterways[C]//IECON 2022–48th Annual Conference of the IEEE Industrial Electronics Society. IEEE, 2022: 1-6.